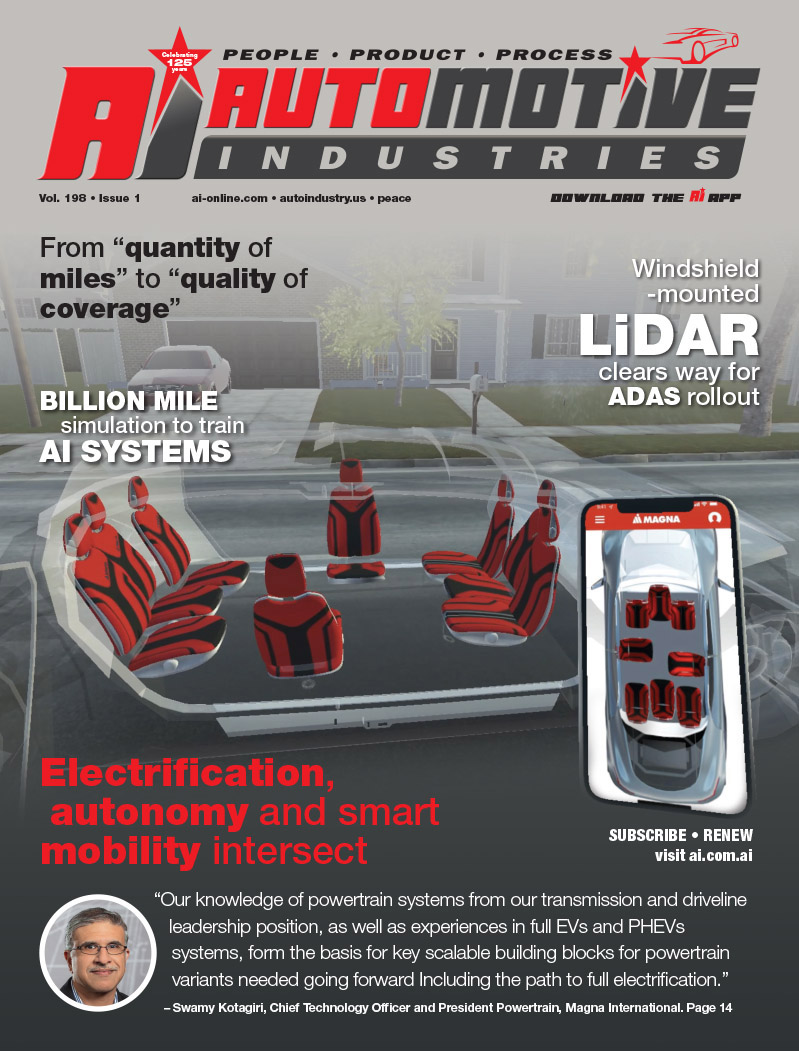
How autonomous vehicles workÂ
Autonomous vehicles, otherwise known as uninhabited autonomous vehicles (UAV), autopilot vehicles, driverless cars, auto-drive cars, or automated/autonomously guided vehicles (AGV), are regarded as intelligent machines. Alan Turing in his classic 1950 paper, Computing Machinery and Intelligence, asked âCan Machines Think?â. Of course, this begs the question of what it means to think. Yet, if we set apart mental activity not done by humans and by an artificial device but upon which we rely to take action that normally would follow from our own thoughts, then we have at least some elements of artificial thinking. Recall (memory), computation, and pattern recognition are activities that can be done better with computers than by humans, and we traditionally have regarded these as thinking. Navigating a car can be done entirely by a human thinking but often better by a computer, simply because of the precision.Â
A system of systems â design goalsÂ
No one component of a system that drives such a vehicle is responsible but a collection of components.Â
Since the 1950s rapid development of âintelligent systemsâ has occurred, including tire monitoring, human-machine interfaces, assisted steering, brake management, navigation, and now semi-autonomous and autonomous driving. Numerous and different systems have been deployed, giving drivers directions through the global positioning system (GPS) as to the best routes both audibly and by text [2].
The only step remaining was to coordinate the cars with each other, and full automation appeared to be the optimal way of doing this. In 1997, the California Partners for Advanced Transit and Highways 2006 (PATH) demonstrated that a row of cars could be driven automatically down the highway at normal speeds with close spacing [3]
A very wide network and interconnected solutions is needed, however. Vehicles can be treated as parts of a large organism when viewed all at once, rather than as individuals in isolated personal units. That means there has to be an integrated transport system that coordinates traffic flow in order to make the system work optimally. Drivers may have the choice to override the recommendations of a car’s computer, but such could cause grave complications the integrated system might not be able to handle, as the change in one component, a car, may well affect how the others will navigate. Perhaps research from swarm theory will be able to guide us, but at this stage, an integrated transit control system operates not unlike a packet of information being routed over a communications network by sophisticated management software or like a power grid, where one disruption can cause the whole network to go down. How much independence a driver should have will have to be assessed in such contexts. People may perforce have less of a choice about being in total charge about where and when they will navigate. We see the emergence of mesh networks, where cars communicate with each other, much in the same way people do by walking in crowded spaces, keeping spaced by selecting the most appropriate routes. For traffic management, it may be a matter of necessity to have a person entering a crowded area being switched to a network and driven according to the needs computed by the traffic management software. Such is not much different than an air traffic control system, where each airliner lands in turn, given the configuration of airport traffic. High Occupancy Vehicle (HOV) lanes are a precursor to what we may expect in selective autonomous traffic control. At this juncture, it appears almost necessary to have a fully autonomous vehicle that can respond to such a system. How feasible is this, and how would such work?Â
Want to learn more about current technologies and developments automotive navigation?
Visit our Download Centre for more articles, white papers and interviews:Â
http://bit.ly/automotive-navigation Â
References (Subject is indicated by URL â accessed 2 August 2011)
[1] http://www2.ece.ohio-state.edu/citr/Demo97/osu-av.html
[2] http://reviews.cnet.com/best-gps/
[3] https://docs.google.com/viewer?url=http://www.ece.gatech.edu/~magnus/Papers/UGCPaper.pdf&embedded=true&chrome=true
[4] https://docs.google.com/viewer?url=http://www.ece.gatech.edu/~magnus/Papers/UGCPaper.pdf&embedded=true&chrome=true
Resources (Subject is indicated by URL â accessed 2 August 2011)
http://e2af.com/trend/080212.shtml
http://www2.ece.ohio-state.edu/citr/Demo97/osu-av.html
http://wn.com/automobile_navigation_system – compares different types
Autonomous Cars and Society – https://docs.google.com/viewer?url=http://www.wpi.edu/Pubs/E-project/Available/E-project-043007-205701/unrestricted/IQPOVP06B1.pdf&embedded=true&chrome=true
http://www.intempora.com/en/projects/automotive/adas/carsense
http://en.wikipedia.org/wiki/Controller_area_network
http://www.transport-research.info/web/projects/project_details.cfm?id=15260&page=outline
Stanford robot car “Junior” in action, DARPA Urban Challenge
http://www.youtube.com/watch?v=BSS0MZvoltw
http://www2.ece.ohio-state.edu/citr/Demo97/osu-av.html
Transponder use – http://www.mikechiafulio.com/RIDE/system.html
Path selection method – http://ftp.utcluj.ro/pub/docs/imaging/Autonomous_driving/Articole%20sortate/TUBraunschweig/iv980304.pdf
Lane change algorithm for autonomous vehicles via virtual curvature method
– http://findarticles.com/p/articles/mi_m5CYM/is_1_43/ai_n31126282/
Multi-Sensor Lane Finding in Urban Road Networks – https://docs.google.com/viewer?url=http://www.roboticsproceedings.org/rss04/p1.pdf&embedded=true&chrome=true
Automating Acceptance Tests – https://docs.google.com/viewer?url=http://www.se-rwth.de/books/Diss-Berger.pdf&embedded=true&chrome=true
http://www.eetimes.com/design/embedded-internet-design/4214506/Speech-recognition-in-the-car
http://www.bosch-presse.de/presseforum/details.htm?locale=en&txtID=5156
https://docs.google.com/viewer?url=http://www2.selu.edu/Academics/Faculty/ck/paps/JFR.pdf&embedded=true&chrome=true
Collision avoidance – http://e2af.com/trend/080117.shtml
http://www.intempora.com/en/projects/automotive/adas/carsense
Excellent history summary of UAVs â
http://faculty.washington.edu/jbs/itrans/bishopahs.htm
http://www.ivsource.net/archivep/2000/jul/a000731_carsense.htmlÂ
More Stories
Automotive Industries interview with Johannes Mark, Head of Global Parking Insights, EasyPark Group – Transforming Urban Parking with Light Detection and Ranging Technology
How Secure Parking Technology is Shaping the Future of Urban Development
Steps to Take When Dealing with Insurance Companies After a Car Accident