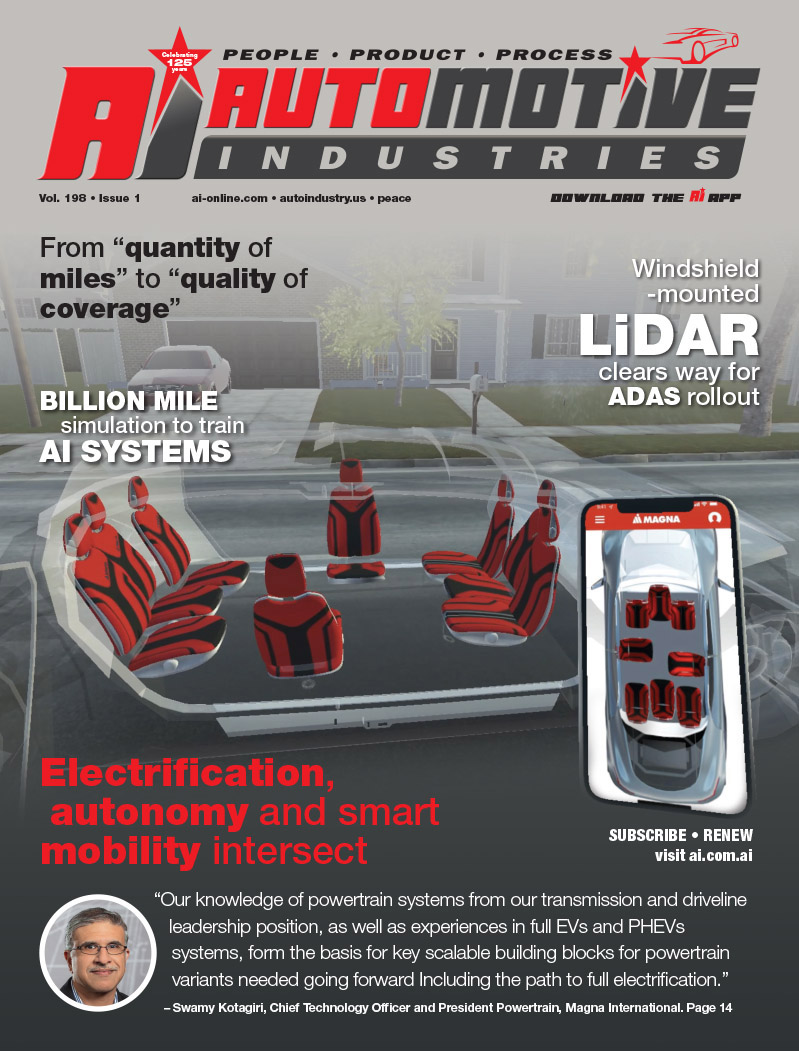
In 2008, according to the National Highway Traffic Safety Administration, 2.3 million automobile crashes occurred at intersections across the United States, resulting in some 7,000 deaths. More than 700 of those fatalities were due to drivers running red lights. But, according to the Insurance Institute for Highway Safety, half of the people killed in such accidents are not the drivers who ran the light, but other drivers, passengers and pedestrians.
In order to reduce the number of accidents at intersections, researchers at MIT have devised an algorithm that predicts when an oncoming car is likely to run a red light.
Based on parameters such as the vehicle’s deceleration and its distance from a light, the group was able to determine which cars were potential “violators” — those likely to cross into an intersection after a light has turned red — and which were “compliant.”
The researchers tested the algorithm on data collected from an intersection in Virginia, finding that it accurately identified potential violators within a couple of seconds of reaching a red light — enough time, according to the researchers, for other drivers at an intersection to be able to react to the threat if alerted. Compared to other efforts to model driving behavior, the MIT algorithm generated fewer false alarms, an important advantage for systems providing guidance to human drivers. The researchers report their findings in a paper that will appear in the journal IEEE Transactions on Intelligent Transportation Systems.
Jonathan How, the Richard Cockburn Maclaurin Professor of Aeronautics and Astronautics at MIT, says “smart” cars of the future may use such algorithms to help drivers anticipate and avoid potential accidents.
“If you had some type of heads-up display for the driver, it might be something where the algorithms are analyzing and saying, ‘We’re concerned,’” says How, who is one of the paper’s authors. “Even though your light might be green, it may recommend you not go, because there are people behaving badly that you may not be aware of.”
How says that in order to implement such warning systems, vehicles would need to be able to “talk” with each other, wirelessly sending and receiving information such as a car’s speed and position data. Such vehicle-to-vehicle (V2V) communication, he says, can potentially improve safety and avoid traffic congestion. Today, the U.S. Department of Transportation (DOT) is exploring V2V technology, along with several major car manufacturers — including Ford Motor Company, which this year has been road-testing prototypes with advanced Wi-Fi and collision-avoidance systems.
“You might have a situation where you get a snowball effect where, much more rapidly than people envisioned, this [V2V] technology may be accepted,” How says.
In the meantime, researchers including How are developing algorithms to analyze vehicle data that would be broadcast via such V2V systems. Georges Aoude SM ’07, PhD ’11, a former student of How’s, designed an algorithm based on a technique that has been successfully applied in many artificial intelligence domains, but is relatively new to the transportation field. This algorithm is able to capture a vehicle’s motion in multiple dimensions using a highly accurate and efficient classifier that can be executed in less than five milliseconds.
Along with colleagues Vishnu Desaraju SM ’10 and Lauren Stephens, an MIT undergraduate, How and Aoude tested the algorithm using an extensive set of traffic data collected at a busy intersection in Christianburg, Va. The intersection was heavily monitored as part of a safety-prediction project sponsored by the DOT. The DOT outfitted the intersection with a number of instruments that tracked vehicle speed and location, as well as when lights turned red.
Aoude and colleagues applied their algorithm to data from more than 15,000 approaching vehicles at the intersection, and found that it was able to correctly identify red-light violators 85 percent of the time — an improvement of 15 to 20 percent over existing algorithms.
The researchers were able to predict, within a couple of seconds, whether a car would run a red light. The researchers actually found a “sweet spot” — one to two seconds in advance of a potential collision — when the algorithm has the highest accuracy and when a driver may still have enough time to react.
Compared to similar safety-prediction technologies, the group found that its algorithm generated fewer false positives. How says this may be due to the algorithm’s ability to analyze multiple parameters. He adds that other algorithms tend to be “skittish,” erring on the side of caution in flagging potential problems, which may itself be a problem when cars are outfitted with such technology.
“The challenge is, you don’t want to be overly pessimistic,” How says. “If you’re too pessimistic, you start reporting there’s a problem when there really isn’t, and then very rapidly, the human’s going to push a button that turns this thing off.”
The researchers are now investigating ways to design a closed-loop system — to give drivers a recommendation of what to do in response to a potential accident — and are also planning to adapt the existing algorithm to air traffic control, to predict the behavior of aircraft.
More Stories
MESSRING completes new crash test facility for Mahindra in India
ROHM Develops an Ultra-Compact MOSFET Featuring Industry-Leading* Low ON-Resistance Ideal for Fast Charging Applications
More than 30 of the top 50 global suppliers have production facilities in Turkey