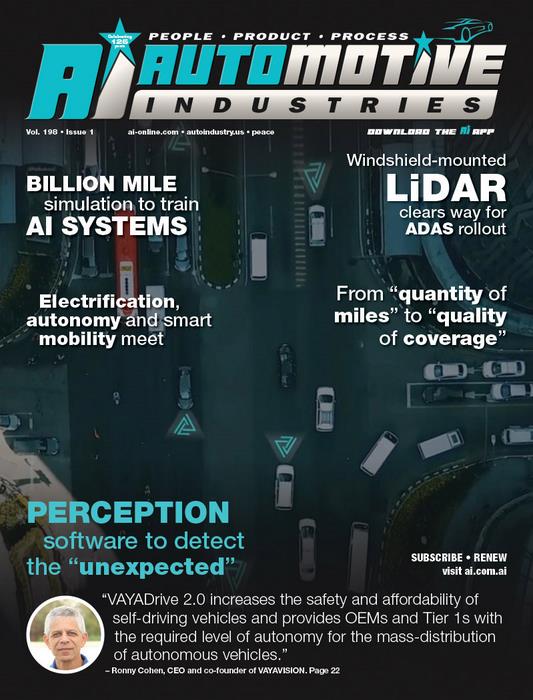
A key challenge facing the industry in the rollout of selfdriving vehicles is the detection of “unexpected” objects.
Roads are full of “unexpected” objects that are absent from training data sets, even when those sets are captured while travelling millions of kilometers. Thus, systems that are mainly based on deep neural networks fail to detect the “unexpected”. In response to this Israeli company VAYAVISION has introduced VAYADrive 2.0, an autonomous vehicle perception software engine that fuses raw sensor data together with artificial intelligence tools to create an accurate 3D environmental model of the area around autonomous vehicles.
“Most current generation autonomous driving solutions are based on ‘Object Fusion’ architecture, in which each sensor registers an independent object, and then must reconcile which data is correct,” said Youval Nehmadi, CTO and cofounder of VAYAVISION. “This provides inaccurate detections and results in a high rate of false alarms. The industry has recognized that to reach the required levels of safety, more advanced perception paradigms are needed – such as raw data fusion.
” VAYAVISION specializes in combining the environmental data received by the self-driving car from different sources — such as LiDAR (light detection and ranging), radar, and camera — to create a comprehensive model of what’s going on around the vehicle. “This launch (of the system) marks the beginning of a new era in autonomous vehicles, bringing to market an AV (autonomous vehicle) perception software based on raw data fusion,” says Ronny Cohen, CEO and co-founder of VAYAVISION.
“VAYADrive 2.0 increases the safety and affordability of self-driving vehicles and provides OEMs and Tier 1s with the required level of autonomy for the mass-distribution of autonomous vehicles.” The company’s system generates precise 3D modelling of the vehicle’s environment using a fusion of raw data from multiple sensors; LiDAR, cameras and RADAR.
Integration of deep understanding of the data, machine vision algorithms and deep neural networks provides better cognition essential for SAE level 3 or higher autonomous cars. The result is fewer missed detections and less false alarms by the auto-piloting platform, says the company.
VAYAVISION says no single type of sensor can be relied on to detect objects in the road. Cameras don’t see depth, and distance sensors such as LiDAR and Radar possess very low resolution. VAYADrive 2.0 up-samples low-res samples from distance sensors and assigns distance information to every pixel in the high-resolution camera image. This allows autonomous vehicles to receive crucial information on an object’s size and shape, to separate every small obstacle on the road, and to accurately define the shapes of vehicles, humans, and other objects on the road.
In 2018 VAYAVISION received US$ 8 million funding from Viola Ventures, Mizmaa Ventures, and OurCrowd, together with strategic investment from Mitsubishi UFJ Capital and LG Electronics. The company used the capital injection for marketing efforts and to focus on building partnerships across the world. The launch of VAYADrive 2.0, is expected to give a fillip to the company as more automotive OEMs scout for effective autonomous vehicle technologies.
Automotive Industries (AI) asked Cohen how VAYADrive 2.0 will help autonomous vehicles ‘up-sample’ from AV levels 3 and 4, to level 5 Cohen: For Level 5, perfect environmental perception is required – one that has detection rates of 100% and close to zero false alarms. The technology behind VAYADrive 2.0 is exactly what is required to “up-sample” and provide the required level of performance by taking a different approach from the rest of the industry. With our raw data fusion and up-sampling technology, we create an accurate HD 3D RGBd model that is the base for DNN (deep neural network) and non-DNN detection algorithms that run in parallel and achieve the desired Level 5 performance levels.
AI: What is the real breakthrough? Cohen: The technology is able to detect small obstacles and “unexpected” objects. It has inherent redundancy and addresses the need for safety. As a result, vehicle perception can now be much better and more accurate than human driver perception.
AI: How does the “brute force” solution of current sensing systems compare with one such as yours? Cohen: People are trying brute force solutions that use multiple costly sensors, and then process each sensor separately before fusing the data together the processing results. This process uses a lot of computing power, thus incurring high solution prices. VAYAVISION has analyzed the underlying scenarios and issues and used its extensive collective background in physics, AI, computer vision, and mathematics to come up with a solution based on raw data fusion and up-sampling that can use low-cost sensors and minimal computation power, while reliable and accurate.
AI: How successful was VAYAVISION’s participation in this year’s CES 2019? Cohen: CES 2019 was a significant time for both VAYAVISION and the AV industry, as we released our first product, VAYADrive 2.0, which is the first AV perception software with full environmental model to use raw data fusion and up-sampling. We received a lot of interest and appreciation from the industry for our innovative technology, which exhibits high performance rates. People were amazed at VAYADrive 2.0’s ability to detect small obstacles no matter their shape or location – something that is unattainable when relying on the DNN (which requires a lot of training that is inadequate regardless, as it is virtually impossible to train for all objects). The show generated for us many leads and opened the door for many exciting opportunities.
AI then asked Nehmadi how close cars are to Level 5 of autonomous driving. Nehmadi: We do not yet see the level of maturity required for level 5 autonomous driving. However, we do see that restricted Level4/Level5 driverless public transportation services will happen soon, and are in fact already being announced. Specifically, this includes shuttles, robo-taxis and the like that move along predetermined routes at low speeds, thus circumventing maturity issues and meeting required safety levels. We trust that our technology can bridge the gap, allowing for more use cases and expanding the use of Level 4 and 5.
AI: How does your technology “detect the unexpected”? Nehmadi: Our approach incorporates inherent redundancies that comply with functional safety. We have two sets of algorithms executed in parallel: One is AI-based (DNN that requires training) and the other is Non-DNN. A good example of this approach is the detection of “unexpected” objects that were not in the training set (of the DNN), thus preventing DNNs from detecting them should they appear on the road “unexpectedly.” Our non-DNN approach uses an accurate and dense 3D RGB-D model to detect and locate objects, and identify if they occupy the free space through which the AV will drive. This is critical for safe autonomous driving. The solution tolerates automatically and continuously malfunctioning sensors or temporarily missing data from one of the sensors. Since the solution fuses together raw data from sensors and tracks the data over time, it can tolerate failures. Traditional sensor processing technologies first process each sensor separately and then fuse the object data together, rendering them incapable of “seeing the big picture.” It is harder for AVs to make decisions when multiple sensors are processed separately, as this may produce different and contrasting outputs on whether free and drivable space exists or not.
AI: How does VAYAVISION plan to expand its global footprint? Nehmadi: VAYAVISION is using its funding to expand its international presence, work with additional global OEMs and Tier 1s, and roll out its product to the market.
More Stories
MESSRING completes new crash test facility for Mahindra in India
ROHM Develops an Ultra-Compact MOSFET Featuring Industry-Leading* Low ON-Resistance Ideal for Fast Charging Applications
More than 30 of the top 50 global suppliers have production facilities in Turkey