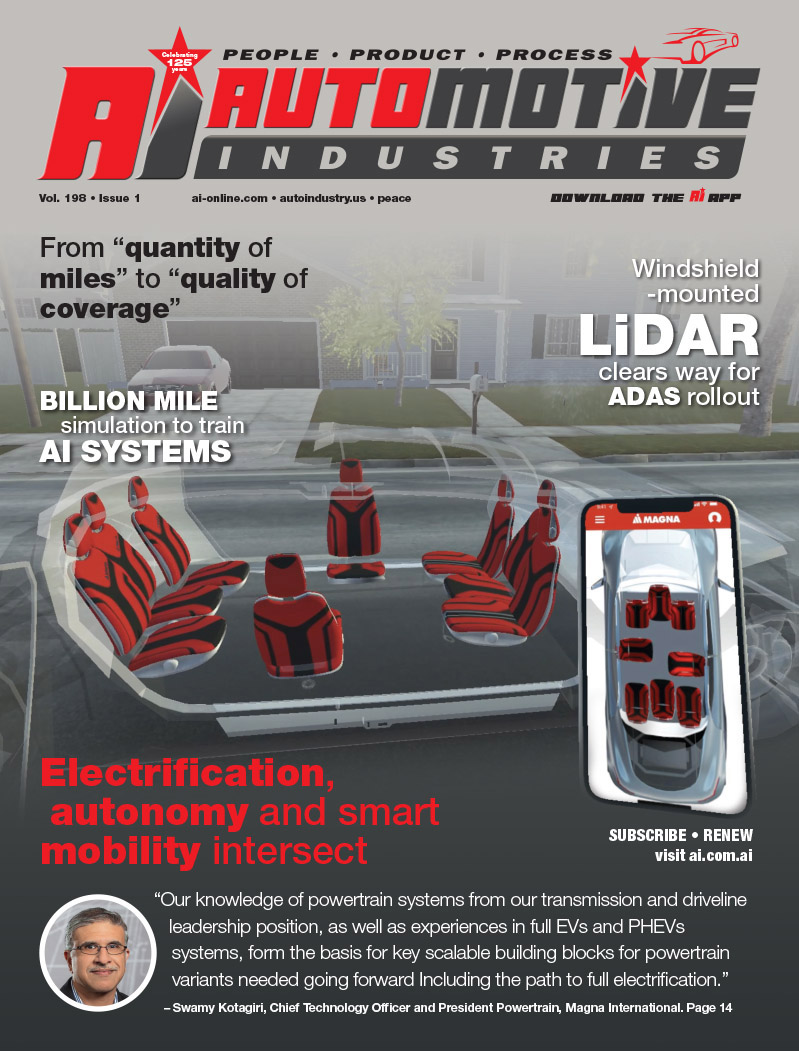
According to the National Highway Traffic Safety Administration or NHTSA, there were nearly 5 and a half million vehicular crashes in 2010 – many of them at traffic intersections. A few years back, the NHTSA along with General Motors conducted an Automotive Collision System Field Operational Test (ACAS FOT) which ran for five years. A problem that kept cropping up was false alarms. Nearly half the alerts were due to stationary objects on the roads being detected by the radar and erroneously classified as threats to the host vehicle.
Now, MIT researchers believe they may have the answer to the problem of false alarms that face many automotive collision avoidance systems. The institute’s researchers have developed an algorithm that could potentially slash the number of car crashes at traffic intersections. The algorithm predicts when an oncoming car is likely to jump a red light. Based on parameters such as the vehicle’s deceleration and its distance from a light, the group was able to determine which cars were potential ‘violators’ — those likely to cross into an intersection after a light has turned red — and which were ‘compliant’.
Jonathan How, the Richard Cockburn Maclaurin Professor of Aeronautics and Astronautics at MIT led the team of researchers tested the algorithm on data collected from an intersection in Virginia, finding that it accurately identified potential violators within a couple of seconds of reaching a red light — enough time, according to the researchers, for other drivers at an intersection to be able to react to the threat if alerted. Compared to other efforts to model driving behavior, the MIT algorithm generated fewer false alarms, an important advantage for systems providing guidance to human drivers. This is because if the system is too cautious, drivers could be tempted to switch off the collision avoidance system.
“If you had some type of heads-up display for the driver, it might be something where the algorithms are analyzing and saying, ‘We’re concerned’. Even though your light might be green, it may recommend you not go, because there are people behaving badly that you may not be aware of,” said How.
He says that in order to implement such warning systems, vehicles would need to be able to talk with each other, wirelessly sending and receiving information such as a car’s speed and position data. Such vehicle-to-vehicle (V2V) communication, How says, can potentially improve safety and avoid traffic congestion. Today, the US Department of Transportation (DOT) is exploring V2V technology, along with several major car manufacturers — including Ford Motor Company, which this year has been road-testing prototypes with advanced Wi-Fi and collision-avoidance systems.
According to Ford, 81 percent of accidents in which alcohol isn’t related are due to hazards that may be predicted and warned of through V2V technology. Ford’s goal is to reduce and hopefully eliminate a large chunk of those 4.3 million accidents that occur each year. In addition to providing an extra level of safety for the drivers, V2V technology could also reduce gas wasted due to traffic delays. In 2009, the Texas Transportation Institute reported that 3.9 billion gallons of gas were wasted due to traffic issues. Ford hopes that its V2V technology will not only keep the drivers safe, but to reduce the hundreds of dollars that commuters waste due to traffic.
Ford’s V2V technology works via an independent short-range WiFi system broadcasted on a secure channel allocated by the FCC. Ford is confident that the technology works better than radar safety systems due to the fact that it allows a full 360-degree range around the vehicle, with or without a direct line of sight. This technology would be efficient and powerful in predicting oncoming collisions with unseen vehicles, sudden stops and any changes in traffic that may occur unexpectedly. Ford expects its future models that will be launched in 2012 and 2013 to include its V2V technology.
“The day is not far off when our vehicles will operate like mobile devices with four wheels, constantly exchanging information and communicating with our environment to do things like shorten commute times, improve fuel economy and generally help us more easily navigate life on the road,” said Paul Mascarenas, Ford’s vice president of research and engineering.
How added: “You might have a situation where you get a snowball effect where, much more rapidly than people envisioned, this [V2V] technology may be accepted.”
Automotive Industries spoke to Professor Jonathan How, the Richard Cockburn Maclaurin Professor of Aeronautics and Astronautics at MIT.
AI: Tell us a little about the process of developing the algorithm and later applying it to traffic situations.
The idea for this project started with our participation in the DARPA Urban Challenge in 2007. I was in charge of the planning and control of the MIT Team (with John Leonard, Seth Teller, and Emilio Frazzoli) which placed fourth in the race in November of that year. While we were very happy with the performance of the planning system that we had developed, a post-analysis of the race indicated that several components needed to be improved. For example, one issue was that our planner had, by necessity because the time-scales of the development were so short, relied on relatively simple models of the motions of the other cars on the course. Furthermore, the models of this behavior were rigid – there was no means to update in real-time based on recent experiences. Thus the goal of this project was to develop a threat assessment module for a human driven car that could learn and adapt these models of the possible behaviors of the other vehicles on the road. In discussions with researchers at Ford, it was clear that an intersection was a good scenario to consider because of the relatively large number of accidents and deaths related to them. Georges Aoude, the former PhD student that performed this research was able to obtain some data that was collected as part of the Cooperative Intersection Collision Avoidance System for Violations (CICAS-V) project, which was then analyzed for this paper.
AI: What makes MIT’s traffic collision avoidance algorithm more effective than other similar algorithms developed by others, including automotive OEMs?
The paper describes two algorithms that were developed by Dr. Aoude. Both perform well in the analysis, but the better of the two combines an algorithm called support vector machines (SVMs) with a Bayesian filter. SVMs have proven to be particularly effective classification algorithms in many other domains, and this work develops a novel architecture that enables it to perform well on the driver behavior classification problem.
The SVM extracts the relevant features from the sensor observations and then outputs a single classification (violator vs. compliant). The Bayesian filter uses this information to update the estimate of the probability that the driver will be red-light compliant. An important distinction between this work and much of the driver classification literature is the flexibility that exists within the SVM to combine many metrics and parameters in the same classification model.
AI: What makes your automotive crash avoidance algorithm less prone to false alarms?
Again, the key to the algorithm is that it blends in many factors in the classification of good or bad driving behavior as cars approach red lights. The technique uses the SVM algorithm to learn the right blending of these factors from the available data. The classification out of the SVM is then filtered to avoid being overly responsive to noise in the real time measurements.
AI: How have automotive OEMs reacted to MIT’s collision avoidance algorithm? Please give us details.
The initial feedback has been positive, but we have not definitively decided on any paths forward at this time.
AI: What impact in your opinion, will this automotive crash avoidance system have on bringing down the number of accidents?
Our hope is that it will increase the situational awareness of the drivers so that they can make better-informed decisions about how to proceed. This should, for example, help avoid situations in which slight distractions or a slightly blocked view have significant consequences because they lead to crashes.
AI: Do you see a time when such safety systems become mandatory for all vehicles?
I think that would make sense, but it really is something that others must determine.
AI: What other applications do you see for this particular algorithm?
Our current work is applying similar technologies to collision avoidance algorithms between aircraft, possibly including unmanned vehicles (UAVs).
More Stories
Cybord warns of dangers of the stability illusion
How Modern Power Management Enhances Connected Fleet Tech
Avery Dennison PSA tapes support rapid evolution of EV batteries